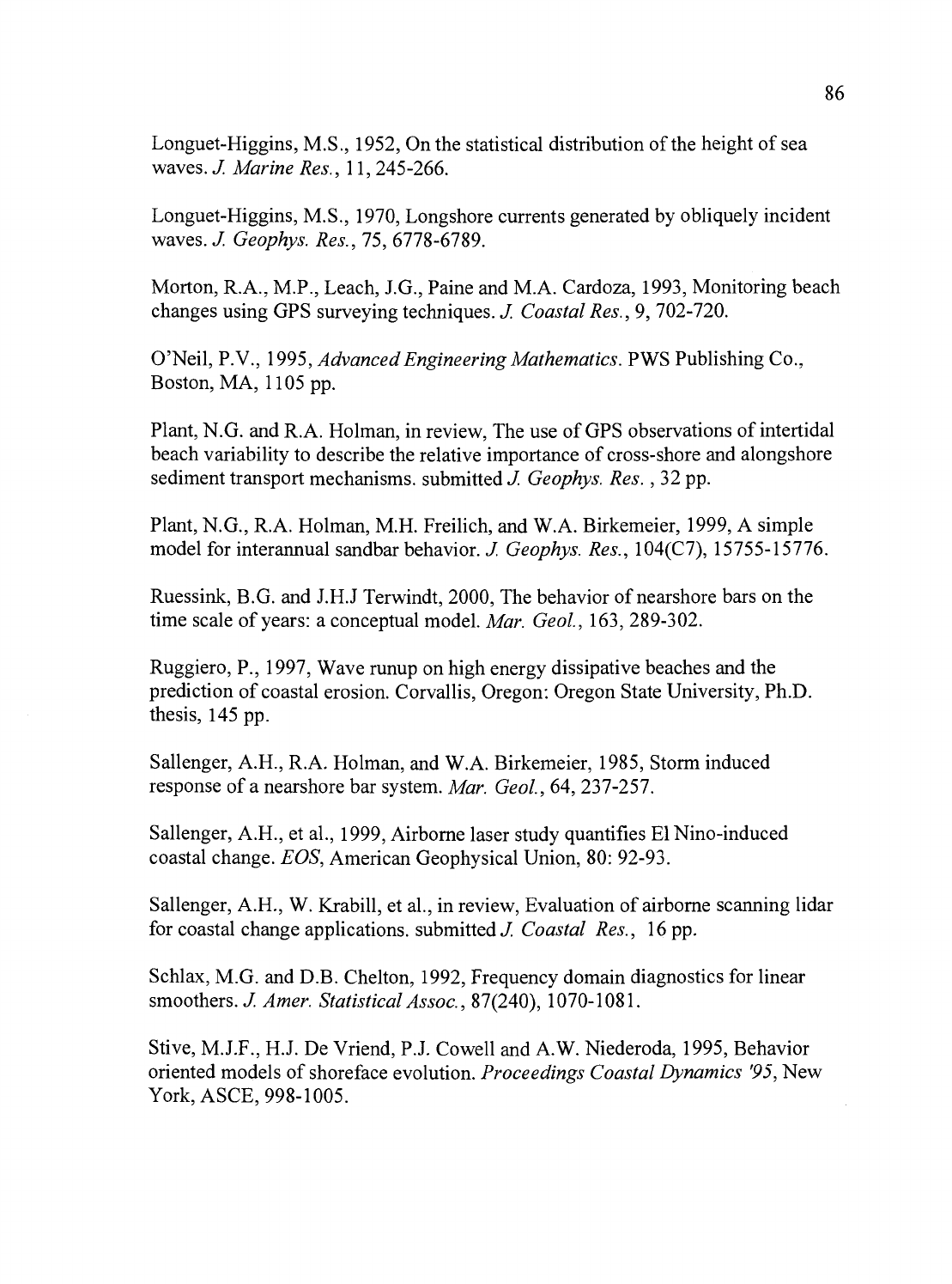
Longuet-Higgins, M.S., 1952, On the statistical distribution of the height of sea
waves. J. Marine Res., 11, 245-266.
Longuet-Higgins, M.S., 1970, Longshore currents generated by obliquely incident
waves. J Geophys. Res., 75, 6778-6789.
Morton, R.A., M.P., Leach, J.G., Paine and M.A. Cardoza, 1993, Monitoring beach
changes using GPS surveying techniques. I Coastal Res., 9, 702-720.
O'Neil, P.V., 1995, Advanced Engineering Mathematics. PWS Publishing Co.,
Boston, MA, 1105
pp.
Plant, N.G. and R.A. Holman, in review, The use of GPS observations of intertidal
beach variability to describe the relative importance of cross-shore and alongshore
sediment transport mechanisms. submitted I Geophys. Res. ,
32 pp.
Plant, N.G., R.A. Holman, M.H. Freilich, and W.A. Birkemeier, 1999, A simple
model for interannual sandbar behavior. I Geophys. Res., 1 04(C7), 15755-15776.
Ruessink, B.G. and J.H.J Terwindt, 2000, The behavior of nearshore bars on the
time scale of years: a conceptual model. Mar. Geol., 163, 289-302.
Ruggiero, P., 1997, Wave runup on high energy dissipative beaches and the
prediction of coastal erosion. Corvallis, Oregon: Oregon State University, Ph.D.
thesis, 145 pp.
Sallenger, A.H., R.A. Holman, and W.A. Birkemeier, 1985, Storm induced
response of a nearshore bar system. Mar. Geol., 64, 237-257.
Sallenger, A.H., et al., 1999, Airborne laser study quantifies El Nino-induced
coastal change. EOS, American Geophysical Union, 80: 92-93.
Sallenger, A.H., W. Krabill, et al., in review, Evaluation of airborne scanning lidar
for coastal change applications, submitted I Coastal Res., 16
pp.
Schlax, M.G. and D.B. Chelton, 1992, Frequency domain diagnostics for linear
smoothers. I Amer. Statistical Assoc., 87(240), 1070-1081.
Stive, M.J.F., H.J. De Vriend, P.J. Cowell and A.W. Niederoda, 1995, Behavior
oriented models of shoreface evolution. Proceedings Coastal Dynamics '95, New
York, ASCE, 998-1005.